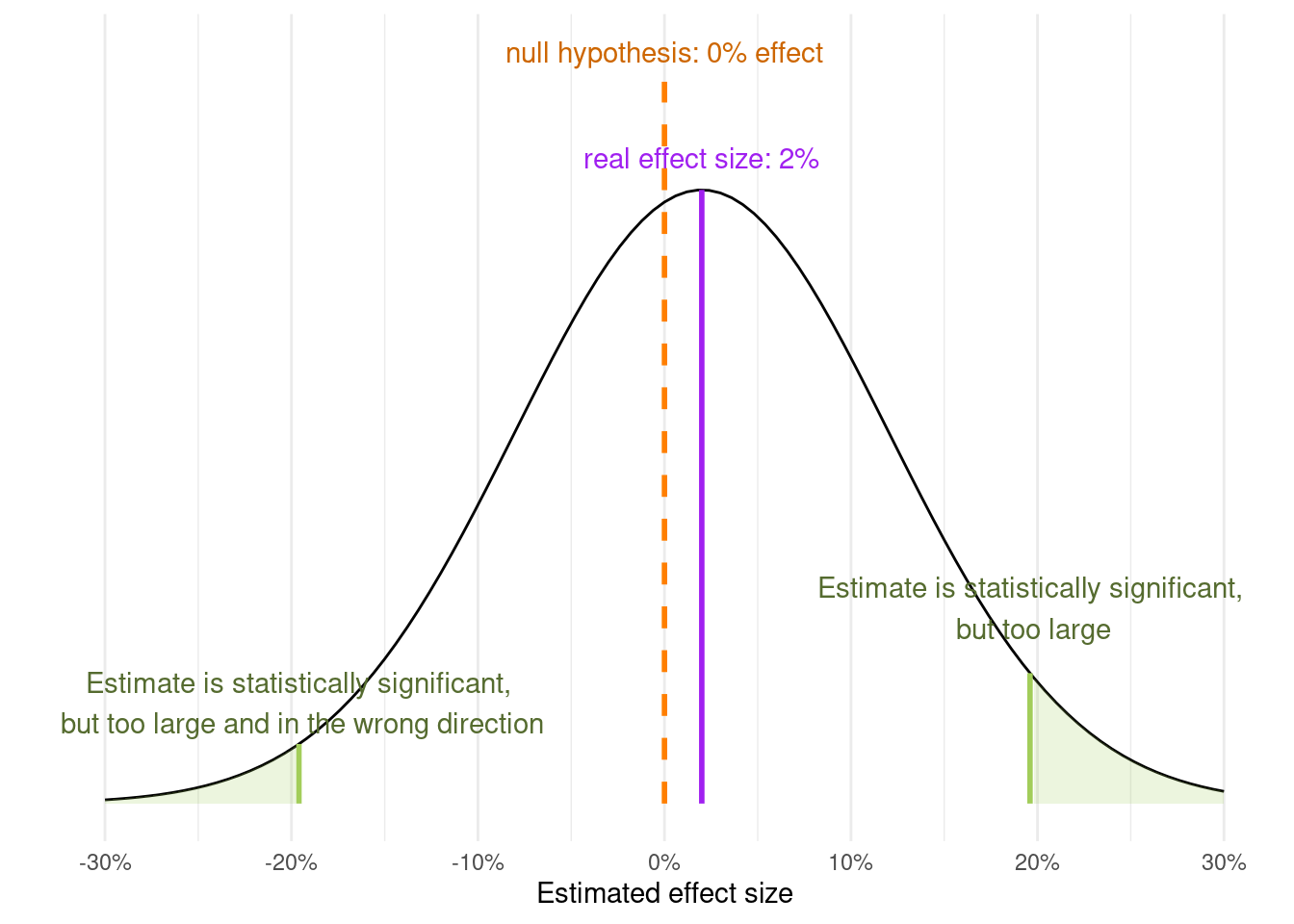
11 Underpowered Studies
We sometimes refer to studies as being “low-powered” or “underpowered.” What we mean by this is that the expected effect size is small in relation to the variation (e.g. standard error) of the measurement. Low power in studies might result from any number of reasons, such as the experiment involving too few experimental units (e.g. individual subjects), or from measurements having relatively high variability due to the effect of noisy nuisance variables.
Underpowered studies may not give a statistically significant result even when a relatively large effect is found in the experiment. Worse still, because a very large effect may be required to reach statistical significance, any “positive” result (rejecting the null hypothesis) is likely to be a statistical outlier and not reflect the true effect size.
Even experienced scientists with many publications and long track records of research funding may never have received any formal training in experimental design or statistics. You will sometimes hear claims being made along the lines of:
“if the effects are large enough to be seen in a small study they must be real large effects”
Unfortunately, this is not true. “Statistically significant” results from underpowered studies are highly likely to be exaggerated, and maybe even suggest that the effect acts in the wrong direction! (Figure 11.1)
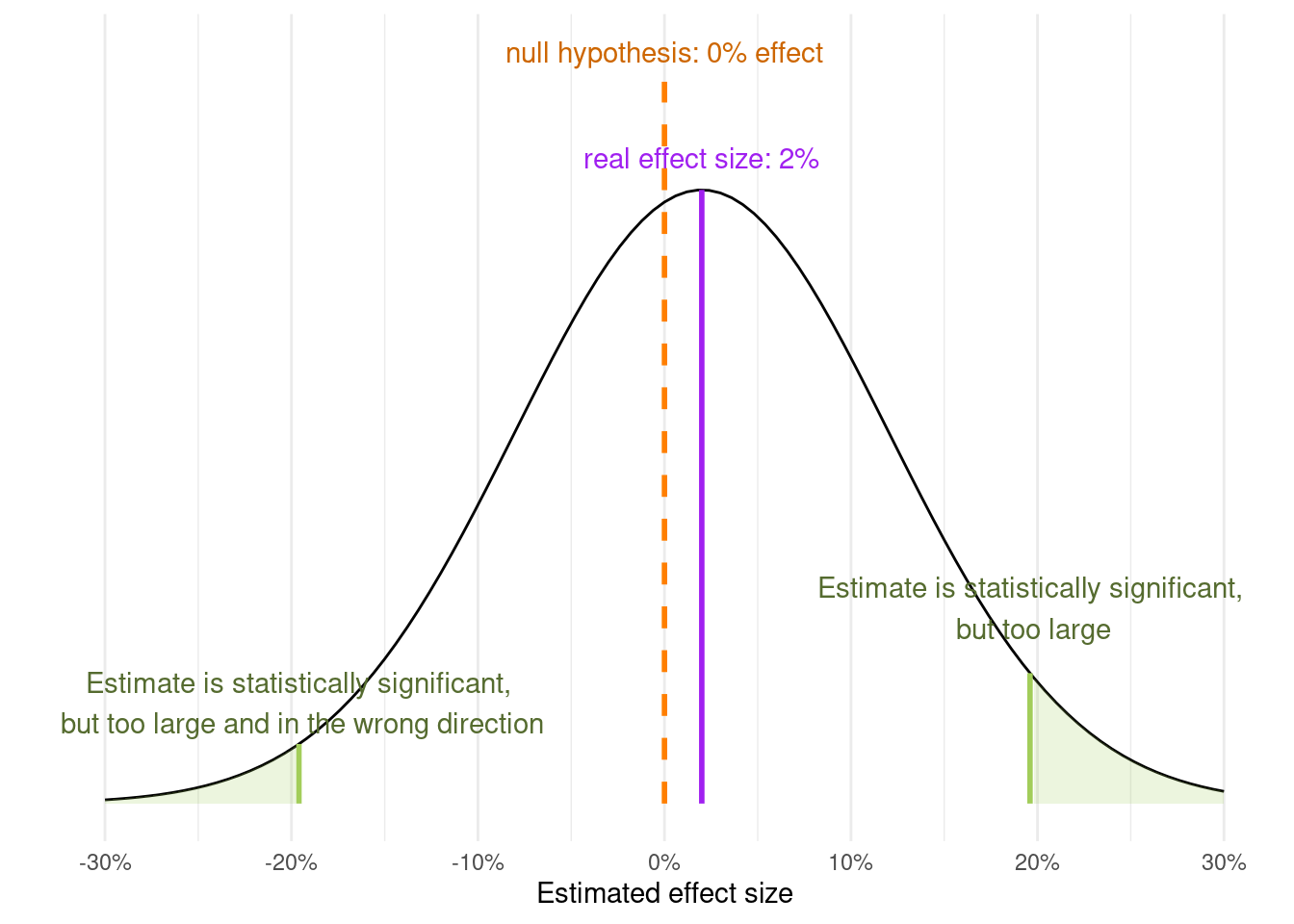
There are three broad strategies available to us, as researchers, to increase the statistical power of an experiment:
- Reduce the variability in the experiment
- this typically means controlling for nuisance effects that cause variation in experimental units, or improving the way we measure outcome variables
- Increase the number of experimental units
- when we increase the number of experimental units, we reduce the uncertainty in our estimate of error of the mean
- Increase the effect size
- this might not always be under researcher control but might be modulated by, say, changing the concentration of an administered drug